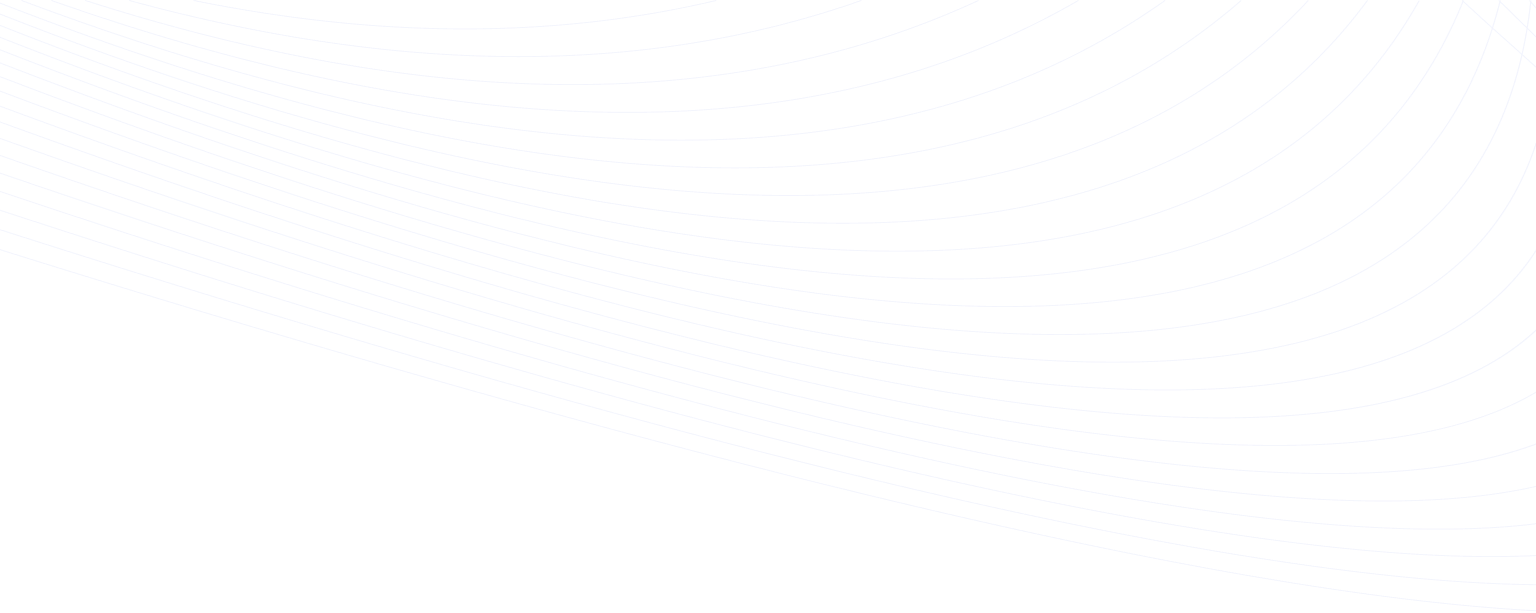
With data technology evolving at an alarming rate, it can seem impossible to keep track of all the latest trends in data storage and architecture. Two hot topics today are the data lakehouse and data mesh. If you’re looking for straightforward information on what these terms actually mean and how they differ, you’re not alone.
How did we get to such a complex data storage landscape?
Until the dawn of big data, data warehouses were the optimal solution to store structured data for specific business operations. But when businesses started growing with unstructured data, it didn’t suit the data warehouse model. This led to the development of a data lake where raw data is stored in its original format.
Data Lakehouse and Data Mesh are the latest data storage management architectures. Whereas the former is an advanced approach to traditional built from centralized data systems, the latter is a modern approach to decentralized data.
Let’s dive deep into both architectures and understand the difference between data lakehouse and data mesh. In less than 10 minutes, not only will you know why there’s such a buzz around these architectures, but you’ll be able to list five specific ways in which they differ.
What is a Data Lakehouse?
Data lakehouse is a hybrid architecture that combines features of both data lake and data warehouse. It supports structured schema and ACID (atomicity, consistency, isolation, and durability) features like those used in the data warehouse. And flexible unstructured data storage features from the data lake. This architecture takes the best features by eliminating downsides from both storage systems.
As a result, it bridges the gap between a data warehouse and a data lake. With data lakehouse, you can store structured, semi-structured, and unstructured raw data to reduce the cost. At the same time, you can use the processed data for streamlined business intelligence processes.
Image Credit: altexsoft
What is a Data Mesh?
Data Mesh is one of the latest approaches to managing data storage systems, introduced by Zhamak Dehghani in 2019. This is a logical architecture for managing data that focuses on the decentralization of data.
Data mesh is one of the modern architectures where each domain captures, processes, organizes, and manages its data. The data is stored and managed with respect to domains like sales, marketing, and more. Each domain owner and team take full control of their data.
This model was introduced to value and access the data easily. It is implemented on its four core principles:
- Domain ownership
- Data as a Product
- Self-serving infrastructure as a platform
- Federated Computational Governance
Image Credit: Datamesh-architecture
For a deep dive into data mesh and how it can transform the organization, see Zhamak’s talk.
Factors that Differentiate Data Lakehouse and Data Mesh
Data Lakehouse vs Data Mesh: Architecture
Data lakehouse is a hybrid architecture. It consists of the following five layers:
- Ingestion Layer: The first layer of data lakehouse architecture is responsible for gathering data from internal or external sources. Then it delivers the collected data to the storage layer.
- Storage Layer: The second layer allows storing structured, semi-structured, and unstructured data in the form of objects in a cost-effective object-store.
- Metadata Layer: This layer provides a unified catalog of Metadata (detailed information describing other data) for all the stored objects.
- API Layer: This layer hosts APIs, enabling end-users to retrieve data quickly.
- Consumption Layer: A number of tools are connected to perform different analytical tasks. This includes Machine Learning (ML), Business Intelligence (BI), Data Science, and reporting.
Data Mesh is a distributed architecture based on its 4 core principles which are:
- Domain Ownership: Domain owners should take ownership of their data. Therefore, end-to-end data should be organized by its respective domain team. This principle sets the accountability on the data ensuring the processed data is contextually accurate.
- Data as Product: This principle aims at producing high-quality data. It states domain teams should provide discoverable, reusable, self-describing, trustworthy, and secure data.
- Self-serve data infrastructure as Platform: This principle gives different domains the autonomy to serve in a self-serving manner reducing repetitive efforts and duplication of data.
- Federated Computational Governance: This principle mandates the consideration of global standards in order to maintain consistency and security. It states an individual set of rules that should be implemented by a specific domain team in their local environment. However, domain teams can have their own set of rules for governance but should still follow global standards.
Data lakehouse is a monolithic architecture focused on optimizing the current data centralization workflows. However, data mesh is a completely different approach to managing the data. Data mesh enables you to move away from the monolithic, centrally managed architecture.
Data Lakehouse vs Data Mesh: Data Storage
With data lakehouse architecture, the data is stored centrally. But, a lakehouse uses inexpensive storage systems to store large amounts of data while ensuring scalability. You can create a lakehouse with AWS S3 buckets or Microsoft Azure Data Lake Storage Gen2 repository. In general, data lakehouse combines both data lake and data warehouse. And as usual, a central team is responsible for storing and organizing data.
However, a data mesh is not about unifying various platforms. Rather, data mesh focuses on the decentralization of data. So, you can still use the data lake and data warehouse separately with the data mesh architecture. With data mesh, you keep storing the data at the same location but just change the way data is collected and governed.
Data Lakehouse vs Data Mesh: Self-Service
In data mesh, the data is decentralized with respect to the domain. Each domain team owns its infrastructure, code, and data. So, data mesh enables self-serve data infrastructure. However, it centralizes the domain-specific data through proper access to metadata. This allows the respective domain team, as well as other domain teams, to access and use these datasets for analytical purposes.
On the other hand, data lakehouse focuses on creating a single source of truth in data lakes. This optimizes data accessibility with respect to other monolithic, centrally managed architectures. While this enhances the self-service capabilities, you still rely on the central team for quality datasets.
Data Lakehouse vs Data Mesh: Data Governance
Data lakehouse follows a uniform way of data governance to manage data quality and compliance across all organizations. This architecture simplifies and improves the quality of data by applying regulations across all organizations' data using the standard interface. This eliminates the need for having separate governance in data lakes and data warehouses.
Data mesh follows the Federated Computational governance principle. This is one of the most important principles of data mesh architecture. This approach keeps the balance between central governance and decentralized data within an organization. The decentralized teams have their own data governance rules, but every team still follows the global or central rules. The centrally governed standards allow interdependence and interoperability across domain teams within organizations. This provides more flexibility over the central-only governance rules of data lakehouse.
Data Lakehouse vs Data Mesh: Administrative Efforts
Data lakehouse reduces the administrative efforts to manage data as you bring both data warehouse and data lake together. So, you build the single source or truth once and access it many times across use cases.
Whereas in data mesh, the domain owner and domain team take full responsibility for managing data. In case, there are eight different domains under one business unit. As per the data mesh principle, the organization would require 8 domain owners to handle the data. This might increase the overall administrative burden, but since the administration is divided across teams, you reap the benefits of having better-quality data.
Conclusion
The main difference between data mesh vs lakehouse is that data lakehouse is a monolithic architecture that follows a centralized approach to the governance of data. But, data mesh is a logical architecture that follows decentralized data management.
Choosing a data lakehouse for small businesses might be a feasible option. As you get to store all data in one place without having to differentiate them domain-wise. But if you have large data sets, require frequently updated analysis, and domain-driven platforms, data mesh would be one of the best options.
However, it is not necessary that you always have to choose between the two—data lakehouse and data mesh. You can also combine both the architecture to enhance data storage and governance.
Whatever architecture you choose, Estuary Flow will help you to streamline data access, reduce costs, and improve overall business decision-making. Try Flow today to get started!
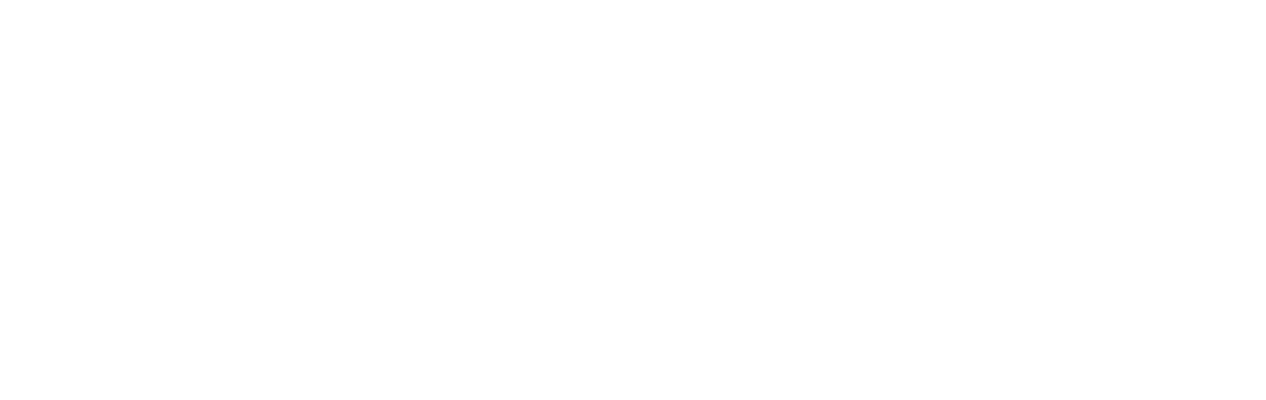
About the author
With over 15 years in data engineering, a seasoned expert in driving growth for early-stage data companies, focusing on strategies that attract customers and users. Extensive writing provides insights to help companies scale efficiently and effectively in an evolving data landscape.
Popular Articles
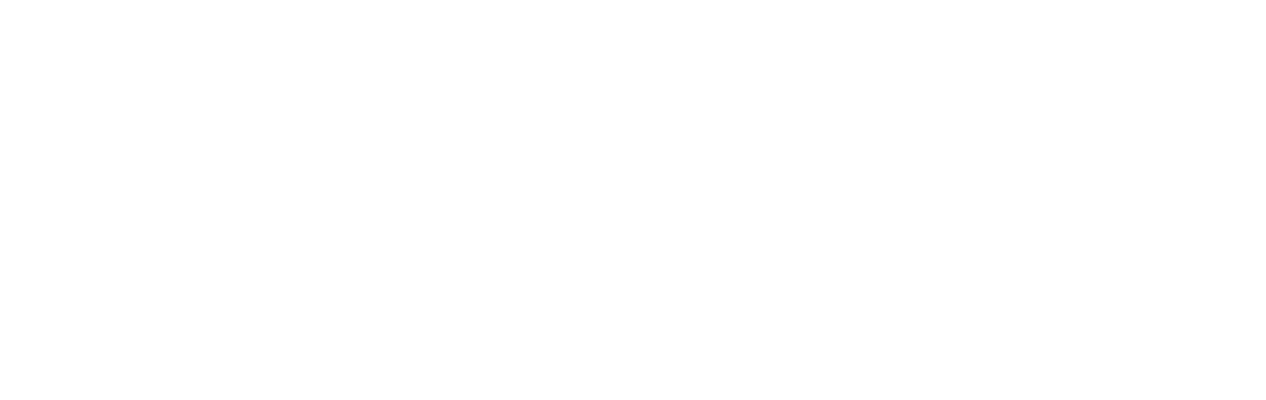